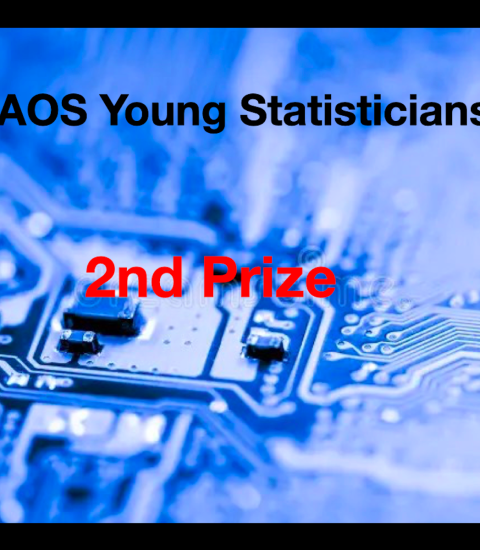
Ryan Covey, works since 2022 as a Data Scientist at the Methodology and Data Science Division of the Australian Bureau of Statistics. He finalized in 2023 his Ph.D. in Econometrics at the Monash University. In 2018 he achieved a Bachelor's degree of Electronical and Computer Systems Engineering and a Bachelor of Commerce.
Dear Ryan, please accept my warmest congratulations on this prize
Ryan: Thank you Pieter! And thanks for inviting me to speak with you today.
SJIAOS: As a starter for this interview, can you tell us why did you chose to join the Australian Bureau of Statistics as a fresh Econometrics Ph.D. from Monash University?
Ryan: I chose to join the Methodology and Data Science Division (MDSD) of the Australian Bureau of Statistics (ABS) for a couple of reasons. I think that the division is a natural place for someone with an econometrics background because of the modeling work that is done in MDSD and the need for standard errors when designing surveys. Depending on the research topic, an econometrics PhD often equips you with the tools to derive and compute valid standard errors across a wide range of scenarios. I was also interested in how data can be produced (e.g., via probability sampling) and how we can go about obtaining data in an optimal way. In econometrics, we would usually treat the data as given, so I was excited about this new application of the econometrics toolkit.
SJIAOS: Your prize-winning manuscript is on the integration of Big Data and survey data. Can you tell us a bit more about the motivation for this specific research?
Ryan: When a National Statistics Office (NSO) like the ABS undertakes a survey, they have control over a substantial proportion of the design and collection process, which can be undertaken to ensure a level of statistical quality. On the other hand, big data is produced elsewhere for other purposes, by organizations that do not necessarily have the same incentives to keep statistical quality in mind. The problem for the NSO is how to use the information contained within big data to produce more accurate statistics when that data might not be a good representation of the target population. In my paper, I propose combining big data and survey data to produce an integrated estimate of the population median and show that this estimate is more accurate than the standard sample median computed using only the survey.
SJIAOS: How were you informed about the YSP prize and what finally stimulated you to write the paper?
Ryan: The YSP prize was circulated throughout MDSD and the ABS through internal newsletters. I had a solution in mind for the problem of integrating big and survey data to estimate the median that drew on some techniques I learned during my Ph.D., and how to take advantage of big data was and is widely regarded in the ABS as an important and tricky problem. The call to action was the push I needed to propose the idea to my manager.
SJIAOS: Did you experience good coaching and support from your team and management?
Ryan: Yes, the coaching and support from my team and management was fantastic. I received feedback from a wide range of people in and beyond MDSD resulting in a substantially improved manuscript.
SJIAOS: The use of Big Data is fast developing and there are many new opportunities for official statistics. In your experience, can you give some examples of ways that you have seen Big Data used in the ABS to support the production of official statistics?
Ryan: I can think of several ways. If the big data set is a complete enumeration of a target population, then it may be appropriate to produce official statistics based on that set alone, if the quality is good enough. Digital surveys in particular can be pre-populated with information retrieved from other sources to reduce provider burden. I am not aware of any official statistics that are currently produced by combining big and survey data in a similar way to what I propose in my YSP paper, so it seems to me that there is potential for further innovation in that area.
SJIAOS: What do you see as the most challenging features of Big Data and the integration with Survey Data and Machine Learning?
Ryan: One of the most challenging features is that the use of big data with survey data can mean that multiple biases need to be corrected (e.g., non-response, measurement error and linkage error), perhaps requiring multiple models. Variance estimation can be difficult in this context where there are multiple sources of uncertainty arising from the estimation of model parameters. This is an issue that I don’t address in the YSP paper, which is concerned only with under- and over-coverage of the big data set and assumes that this is the only source of bias. Variance estimators for statistics produced using machine learning methods are often unavailable, though this is slowly changing and there is interest among researchers for developing these.
SJIAOS: … and what are your expectations for the use of Big Data in the next few years?
Ryan: My hope is that we continue to develop methods for making better use of big data and that we can head towards using big data to conduct more efficient surveys.
SJIAOS: With all the recent new developments in ML, AI, and the ecosystem of data, how do you see the production and dissemination work of the national statistical office being organized in 10-15 years?
Ryan: One area where I see ML influencing the production and dissemination of official statistics is in using natural language processing to match descriptions of companies, work and products to the most appropriate industry, occupation and product code under the relevant standard. We might also see a greater amount of official statistics information disseminated via conversation with large language models like the GPT family that are trained on publically available information, including official statistics.
It was a pleasure to have this interview with you and I wish you success in your further career.
Teaser: Turning big data into accurate official statistics