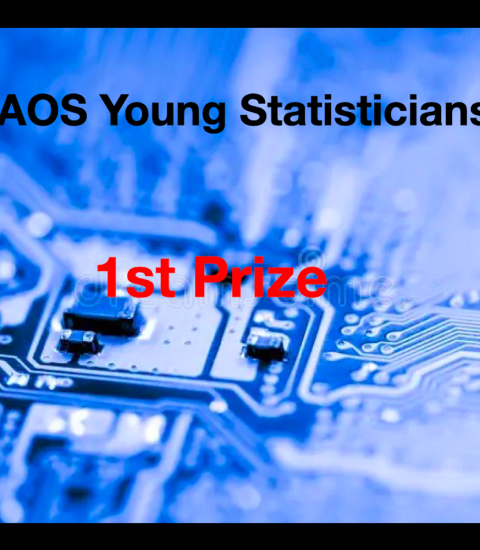
Joanne Yoon, is since June 2022 Lead Data Scientist at Statistics Canada. She started at Statistics Canada in May 2018. She has an M.SC. in Computing Science, Professional Master’s in Big Data at Simon Fraser University (SFU) and a B.Sc in Software Systems at SFU.
Dear Joanne, please accept my warmest congratulations on this prize.
SJIAOS: As a starter for this interview Joanne, can you tell us why you chose to join as a fresh university graduate Statistics Canada?
Joanne: As a proud Canadian, I always wanted to work for the Canadian government and help the government produce services and produce for our citizens. This seemed out of reach for me in Vancouver because I live on the other side of the country from Ottawa. That was until Statistics Canada came to my university's job fair. The director general told me about the potential of applying artificial intelligence (AI) to Canadian data and said "If you love data, work for Statistics Canada which gets data from all over the country". That mesmerized me as a Big Data Master's student. I soon joined Statistics Canada's Data Science Accelerator team as a co-op student. After that, our team quickly grew into its own division in five years due to increasing demand for use cases from the agency.
SJIAOS: Your prize-winning manuscript is on the use of Machine Learning in classifying respondents’ comments from the 2021 Canadian Census of Population. Can you tell us a bit more about the motivation for your research?
Joanne: My day-to-day job is to work on using AI to produce statistical insights on unstructured data, mainly texts.
Subject matters from the census reached out to our team about using AI to classify respondent comments. This project was intended to be an applied project, training a model for the task. As the project progressed, I was intrigued by the data at hand, and I could not resist researching other machine learning (ML) algorithms and evaluating those trained models to overcome class and language imbalances.
The findings and lessons learned from this project helped us when developing text classifiers for other projects. At these moments, I realized the value of the research done on the census respondent comments classification project.
SJIAOS: How were you informed about the YSP prize and what finally stimulated you to write the paper?
Joanne: I was fortunate that the project had great results, and other data analysts were interested in the results of the experiments. With this recognition, I got funding from my branch to spend some time developing this research into a paper. My manager and I wanted to submit it to the IAOS Young Statistician Prize without a doubt, so this new clear goal drove me to write the paper to be up to the standard that the prize withholds.
I started writing the paper to spread my research and findings to others, perhaps another NSO, who may want to train a similar model. This was a healthy and realistic mindset because if I started with the goal to win the prize and be the best submission amongst others throughout the world, I would have made too many edits to go beyond the first section of my paper.
SJIAOS: Machine Learning is fast developing into an important tool for official statistics. How do you personally and in general how in Statscan is ML used to support the production of official statistics of StatCan?
Joanne: This is a question that we research daily to inform our senior management. We keep an eye out for new ML algorithms and models published in the literature and reflect on how they can help Statistics Canada's mission and evaluate if it is ethically responsible and sound. The NLP Centre of Expertise that I chair was also made exactly for this purpose.
Statistics Canada hosts a wide variety of data, including images and texts which I think ML can help Statistics Canada gain more insights on.
SJIAOS: What do you see as the most challenging features of Machine Learning?
Joanne: Getting high-quality data is challenging, especially if I want high-quality labeled data. I was fortunate to have high-quality labeled data for my census respondent comment classification project, but the data was refined through multiple meetings with the subject matter analysts.
.
SJIAOS:… and what are your expectations for Machine Learning in the next few years?
Joanne: I am excited to see how AI will grow in the next years. My team is looking into how we can use generative AI at Statistics Canada. We are yet to apply those new methods to Statistics Canada's data but it would be interesting to see how we can use new methods such as information retrieval chatbots and table-to-text generation to help Canadians better understand the statistical data describing their country's population, resources, economy, society and culture. Also, methods like few-shot learning can help with text classification without requiring subject matter analysts to label a lot of data.
SJIAOS: With all the recent new developments in ML, AI, and the ecosystem of data, how do you see the production and dissemination work of the national statistical office being organized in 10-15 years?
Joanne: We would see more ML models integrated into NSO production systems that sustain or improve the quality and cost-efficiencies of existing methods. I look forward to seeing NSOs leverage ML and statistical methods to utilize administrative data better to increase data timeliness and reduce respondent burden.
SJIAOS: It was a pleasure to meet you and have the opportunity to have this interview done at the margins of the ISI World Statistics Conference, that was held here in your home town Ottawa. Can you share some of your experiences as a Young Statistician from this conference?
Joanne: I was proud that the conference was hosted in Ottawa, and I think the location of this event helped me attend this event even though I was not presenting a session. Throughout the conference, I was amazed by so much interesting statistical research and work going on around the world. I read about it in journals, but to see the statisticians live in front of me and passionately speak about their work and results was a priceless experience. I also appreciated the break times when I could go to the presenters to spark a conversation to see how their methodology could be applied to my work at Statistics Canada. From day one, I was inspired to give a presentation next time.
SJIAOS:…. how did you find the opportunity as a young statistician to participate in such an event?
Joanne: I knew about the event, but I did not feel qualified to attend it. After attending the event, I realized the opportunity lies in the statistical work and not in the age and tenure of the presenter. If my work can benefit from listening to the sessions or if my work fits the criteria to be presented in this event, being young or old never mattered.
The winning manuscript will be published in SJIAOS Vol 39/4 (December 2023).
Thank you for this interview and success in your career.