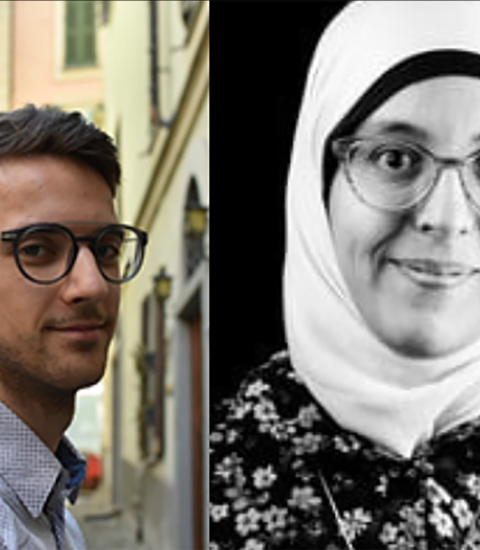
Interview with the winners of the first prize
Firstly we will provide some information about the winners of the award:
Manel Slokom is currently a Postdoc researcher at the National Research Institute for Mathematics and Computer Science in the Netherlands, after working in the Methodology team of Statistics Netherlands from 2021 to 2024. She finalized her Ph.D. in Computer Science from Delft University of Technology in July 2024. She holds a M.Sc. in Data Mining from the University of Nantes, and one in Science and Technologies of Business Intelligence from the University of Tunis.
At the time of submitting his winning manuscript, Jel Vankan had been working with the Microdata Services team at Statistics Netherlands since 2023. He is currently a data scientist at APG (Algemene Pensioen Groep), a pension investment company in the Netherlands. He holds a M.Sc. in Data Science for Decision Making from Maastricht University, and a Bachelor’s in Physics from Eindhoven University.
Dear Manel and Jel, please accept my warmest congratulations on this prize.
SJIAOS: As a starter for this interview, can you tell us why you chose to join Statistics Netherlands?
Manel: When I joined Statistics Netherlands (CBS), I was particularly interested in working on synthetic data and privacy protection, as CBS has numerous use cases and places a strong emphasis on privacy. Over time, I realized that my decision to work at CBS was indeed the right one, as it aligns with my passion for serving society/the public good directly or indirectly.
Jel: I chose to join Statistics Netherlands because they offered a position that perfectly aligned with my interests and expertise. They were looking for someone to conduct research into automated output checking and to develop tools to facilitate this process. What excited me most was the opportunity to create solutions that would significantly assist employees in checking outputs. I was particularly motivated by the potential to streamline the output checking process, enabling researchers to conduct their work more efficiently and with a reduced risk of exposing sensitive information. The combination of innovation and practical application was a perfect fit for my professional aspirations.
SJIAOS: Your prize-winning manuscript is on From COACH to COACH+: Automating Output Checking with Human-in-the-Loop. Can you tell us a bit more about the contents and the motivation for your research?
Manel: COACH stands for COmputer-Assisted Output CHecking. Its goal is to assist human checkers in assessing whether an output, such as a document, table, or plot, is safe to be released. The safety of an output pertains to whether it contains confidential or sensitive information that could be misused. Traditionally, this task is performed manually at CBS by human checkers based on 14 rules of thumb.
In our proof-of-concept work, we propose automating this process with two solutions. First, AOCH, which stands for Automating Output Checking, implements the 14 rules of thumb to check table-based outputs. Second, COACH+ takes a different approach by using multi-model learning. Instead of encoding the rules of thumb, it employs two machine learning (ML) algorithms to predict if an output is safe or unsafe: one ML model is trained on tabular data, and the other is trained on image data.
Additionally, we involve human checkers in the prediction process, a method called human-in-the-loop. Human feedback helps guide and refine the ML models, improving their accuracy and reliability.
Jel: In our manuscript, we explored ways to enhance the traditional output checking process at Statistics Netherlands by integrating automated solutions. Specifically, I focused on developing the Automated Output Checking (AOCH) tool, a desktop application designed to perform data validation according to predefined rules of thumb. This tool helps users quickly identify potentially privacy-revealing information in their data outputs.
AOCH was developed with the user in mind, involving human checkers extensively throughout the development process. This iterative approach of development allowed us to create an intuitive tool with significant flexibility and transparency. Additionally, AOCH served as a baseline for comparing the novel advancements introduced by the COACH+ tool.
SJIAOS: You write that output checking is the process of checking the disclosure risk of research results. What kind of results are you referring to?
Manel and Jel: The term "research results" refers to outputs that contain information or knowledge extracted from input data, i.e., private microdata. These outputs can take various forms, including: tables with aggregated statistics, such as frequency counts, magnitude tables, and percentiles, means etc.; regression analysis coefficients, that is results from statistical models, figures, including histograms, maps, and other visual representations; text files, which may include summaries, interpretations, or detailed descriptions of the analysis.
To explain the process, consider an output as a folder containing mixed files, such as Excel files for tables and PNG or JPEG files for figures. We upload the folder to COACH, which then processes the Excel files using the tabular data ML model and the PNG or JPEG files using the image-based ML model. This allows us to apply predictions to any output, regardless of its type and whether it is internal or external.
SJIAOS: It is a bit difficult for the layman to fully understand how your Output Checking with Human-in-the-Loop works. Could you give us an example of how it could be used for a specific figure in practice, and what would be the disclosure risk?
Manel and Jel: To give an example, suppose that a histogram shows income levels in very fine detail, and there is a bar representing one very high (or very low)-income individual. This could be a disclosure risk because someone familiar with the region might be able to identify this individual. The ML model might flag this as a potential risk, and the human checker would confirm it. Together, they might decide to aggregate the income levels into broader categories to prevent identification. In this way, COACH ensures that even complex outputs like figures and plots are thoroughly checked for disclosure risks, combining the efficiency of ML models with the critical insights of human checkers
SJIAOS: What were the main challenges you experienced in doing this research?
Manel and Jel: The most considerable challenge we faced when building COACH was dealing with the unstructured nature of the input data. The lack of a consistent structure made it difficult for our models to accurately interpret and process the data. The complexity of image files made it challenging for the ML model to distinguish between the different axes within a single figure. These challenges point to the importance of quality data for achieving accurate predictions.
SJIAOS: How were you informed about the YSP prize and what finally stimulated you to write the paper?
Manel: I had a meeting with Peter-Paul de Wolf where I expressed my interest in joining the competition. At that time, I was working on two separate projects: (1) synthetic data and its connection to privacy, and (2) automating output checking with human-in-the-loop. We both decided to go with the second project, COACH, as it was a novel idea and synthetic data had previously won the first prize by Statistics Canada. Also, I strongly believed in the potential of COACH as an idea, method, and application. Additionally, I am usually drawn to challenges, and this opportunity was a perfect fit for my interests.
Jel: Manel had the idea of competing for the YSP, and suggested we work together on this project, which I found a very stimulating challenge.
SJIAOS: Did you experience good coaching and support from your team and management?
Manel: I was part of the Methodology team at Statistics Netherlands. Throughout the project, I collaborated closely with my co-author Jel, who was from Microdata Services and focused on implementing AOCH (hard-coding the 14 rules of thumb). Initially, we engaged with colleagues from Microdata Services, who provided invaluable assistance in familiarizing us with the topic and various types of outputs.
Within the Methodology group, I also worked closely with our co-author Peter-Paul, with whom I regularly brainstormed and discussed design choices across different stages of creation, training, and testing of the machine learning algorithms.
For the human-in-the-loop component, I held intensive meetings with Jel, who manually assessed outputs while I uploaded them to COACH. We compared COACH's decisions with Jel's assessments. If there were discrepancies, I delved deeper into Jel's reasoning and captured his feedback. This feedback was then integrated into COACH, enriching the training data for future predictions.
For the implementation of the COACH front and back end, I received valuable assistance from my sister Malek Slokom, an engineering student.
SJIAOS: What is the role of the Division Data services, Research and Innovation in Statistics Netherlands to which you both belonged when preparing your submission? and how does your work fit with its research programme?
Manel and Jel: Our divisions were supportive and open to innovative projects and ideas. As previously mentioned, our focus with COACH is in the proof-of-concept stage. This phase involves internal exploration and validation, and the tool has not yet been published or officially deployed for operational use. This allows us to refine and optimize COACH based on feedback and testing before wider implementation. Both the COACH project and the synthetic data initiatives are integral parts of the research program at Statistics Netherlands. These projects are recognized for their innovative approaches to addressing challenges in data confidentiality and output checking with human feedback.
SJIAOS: Machine Learning is fast developing into an important tool for official statistics. How do you personally and in general how in Statistics Netherlands is ML used to support the production of official statistics of Statistics Netherlands?
Manel and Jel: The field of Machine learning, trustworthy machine learning and official statistics are rapidly growing. There is a clear need for official statistics to invest more in the use of machine learning technologies. This encompasses not only traditional machine learning methods but also explores the potential of Generative AI, Large Language Models, and other advanced techniques.
However, our stance leans towards a cautious and careful adoption of these technologies. It's essential that we do not merely apply ML models without meticulous consideration of crucial factors such as input data quality, algorithmic transparency, expected outcomes, decision-making rationale, model explainability, and overall model transparency. These elements collectively contribute to ensuring trustworthy machine learning practices.
This being said, let’s recall how historically, we have seen how society initially resisted technology and computers, only to gradually accept them with prudent measures in place. Similarly, we are now navigating a comparable phase with advancements in Artificial Intelligence and Machine Learning. It's imperative that we embrace technological progress while maintaining a responsible and ethical approach. Ultimately, humans should always remain at the forefront of any technology, ensuring that it serves society's best interests.
SJIAOS: What do you see as the most challenging features of Machine Learning?
Manel and Jel: While every step of the machine learning process is important, two components stand out as key to the success of a machine learning model. First and foremost is the input data. Rather than focusing solely on quantity, the emphasis should be on quality data. This includes thorough exploratory data analysis and effective feature engineering to ensure the data is robust and relevant.
Secondly, rigorous evaluation of the ML output is crucial. This includes selecting appropriate metrics to accurately evaluate the performance of the trained model. This evaluation process is key to determining whether the model is ready for deployment into production environments.
Thank you for this interview and best wishes for a successful career.
Interview conducted by Jean-Pierre Cling in July 2024
The winning manuscript will be published in SJIAOS Vol 40/4 (December 2024).
Interview with the winner of the second prize
Firstly, we will provide some information on the winner of second place:
Since June 2020, Alex Imbrogno has been a methodologist in Census Weighting and Estimation at Statistics Canada. He holds an M.Sc. in Statistics, as well as a Bachelor’s in Sociology, both from Carleton University in Ottawa.
Dear Alex, please accept my warmest congratulations on this prize.
SJIAOS: As a starter for this interview Alex, can you tell us why you chose to join Statistics Canada as a fresh university graduate?
Alex: During graduate school, I participated in a 4-month internship as a methodologist at Statistics Canada. It was during this time that I recognized Statistics Canada as a place where I would want to work after graduation. I found that I really enjoyed doing statistical research, which I have been lucky enough to continue pursuing at Statistics Canada. As well, I felt proud to be able to contribute to the numerous statistical programs which positively impact Canadians. The majority of my work tasks are centred around the weighting process for the Census Long-Form Sample Survey.
SJIAOS: Your prize-winning manuscript is on Including Non-Binary Gender in the Calibration Strategy for the Canadian Long-Form Sample Survey Weights. Can you tell us a bit more about the motivation for your research? Did your double training as both a statistician and a sociologist influenced your motivation?
Alex: The initial motivation I had to work on this research was driven by some of my past research working on distributed optimization problems for model fitting. With calibration being framed through the lens of a numerical optimization, I was keen to apply my past experience to solve such a pertinent problem. As I progressed on the research, I began to see more and more the importance of such work on gender diversity, and this further contributed to my motivation. I received a lot of satisfaction from both the methodological and potential societal benefits of the work.
SJIAOS: How were you informed about the YSP prize and what finally stimulated you to write the paper?
Alex: I first heard about the YSP prize from my former co-op supervisor who also received a prize for a paper she submitted. However, it wasn’t until after attending a seminar given by past YSP winners from StatCan that I was motivated to write the paper for my submission. The timing happened to work out that my research was coming to an end just as the YSP prize was being announced. After hearing about the positive experiences of past participants, I was eager to write my submission.
SJIAOS: Did you experience good coaching and support from your team and management?
Alex: Yes, the YSP prize is highly promoted and supported within the Methodology Branch at Statistics Canada. After the 2024 YSP prize was officially launched, a seminar took place where past YSP prize winners (within the agency) shared their experiences and gave advice for submitting a paper. I also had a lot of support from my immediate supervisor, who allowed me the time to work on this project and from many of my colleagues and director who provided valuable comments and feedback on the work.
SJIAOS: Can you give us more details about the Canadian Long-Form Sample Survey and its use for analysis and policies, as this is a very specific to Canada?
Alex: For starters, the Census of Population (including the Long-Form Sample Survey) is the primary source of sociodemographic data for specific population groups such as lone-parent families, indigenous peoples, immigrants, and seniors. As well, Census information has many other important uses in the day-to-day lives of Canadians. Local governments use the census to develop programs and services such as planning for schools and health services. Businesses analyze census data to make critical investment decisions, and social services agencies depend on the census to understand the evolving needs of members of their communities.
For the 2021 Census Program, Canadian households were enumerated using two main types of questionnaires: the short-form questionnaire and the long-form questionnaire. The long-form questionnaire included the same questions as the short form, as well as a set of additional questions aimed at providing a more comprehensive portrait of the Canadian population and Canadian households. For example, some long-form questions ask about: any disabilities or impairments a person may have, first nations status/ancestry, religion and education, to name a few. The long-form questionnaire was distributed to a sample of the population.
SJIAOS: Marginalized groups and specifically gender diversity is an important item in official statistics. How do you personally perceive and in general how in Statistics Canada the topic is on the statistical program?
Alex: In the years prior to the 2021 Census, a data gap on the transgender and non-binary populations was identified. Information on these groups was needed by governments, service providers and other institutions to develop programs and policies that address the concerns and needs of these populations. Because the transgender and non-binary populations are small, the 2021 Census was the statistical tool of choice to get reliable counts at disaggregated levels such as municipalities. Furthermore, since 2018, surveys at Statistics Canada have started collecting and disseminating gender information by default instead of sex at birth. The wealth of statistical information released by these surveys these last few years on various sociodemographic aspects of the Canadian society have helped close the data gap on gender minorities.
SJIAOS: Apart from calibration issues, what are the specific problems that Statistics Canada has to address when conducting surveys on these questions?
Alex: In my view, from a total survey error perspective, to be useful a survey concept must be faithful to the underlying social construct and yet be understandable to the typical respondent when asked about it. Indeed, respondents are not specialists of a given topic (some of which are complex, with many and subtle nuances to it), nor can they be expected to spend a lot of their time and energy reading lengthy descriptions accompanying survey concepts to answer a question properly. For example, a very small proportion of the population could be born with both male and female biological characteristics; these people are often referred to as intersex. While there is a need to learn more about them, it is challenging to come up with a concise yet clear definition that intersex people would relate to, all the while acknowledging that Canadians would be asked in a general survey about a concept most of them have never heard of. It is not as simple as adding an ‘intersex’ category to the sex at birth variable.
SJIAOS: Are you aware of UK Statistics, and maybe other NSOs also asking questions on gender diversity in its census, and how would you compare it with what Statistics Canada does?
Alex: The 2021 Canadian Census of Population was the first international census to collect information on the transgender and non-binary populations. Personally, however, I’m not aware of the gender diversity situation in other NSOs. I understand that Statistics Canada’s experts in this area have been actively collaborating with their colleagues around the world, comparing social contexts, and sharing common challenges and best practices.
SJIAOS: What do you see as the most challenging features of the research on gender diversity issues?
Alex: Even though my IAOS paper touched upon gender, I am not a specialist in the matter. In fact, the coming years may very well take me away from gender-related topics as I’ll be devoting my time and efforts to other methodological challenges. Still, in line with my previous answer about surveying social constructs, I believe it will be challenging to develop survey questions and definitions that are well understood by everyone. In my view, it is important to spend a lot of time and energy on getting those survey concepts right, because the success of the surveying activities certainly depend on those.
SJIAOS: Has your research been used by Statistics Canada for the Long-Form Survey, do you expect it to be for this survey of for others?
Alex: We are currently still in the process of evaluating whether or not the research will be used in the upcoming Census. However, we have received a lot of interest from our internal subject matter analysts in the Social Determinants of Health Section within the Centre for Health Data Integration over the possibility of calibrating on the non-binary group. As well, methodologists in the demography simulation project are interested in using the calibration method to include certain smaller indigenous groups in their calibration constraints.
SJIAOS: Last question, how do you see your work as a young researcher, and would you encourage young colleagues to follow your path?
Alex: I personally feel like at times, it can be overwhelming to be a young person interested in doing statistical research. There are many great papers, statisticians and ideas in existence, and it can feel daunting to step foot into the ocean that is statistical research. I feel the important thing is to work on a problem which you find interesting and important no matter how small it may seem in comparison to the work of others. Everyone has to start somewhere! For me, research has never been a linear process. There may be days/weeks/months without progress and then voila, a solution appears. Sometimes you may work hours at your desk with nothing to show and other times you may have a great idea while out for a walk. Embrace the process!
Thank you for this interview and success in your career.
The winning manuscript will be published in SJIAOS Vol 40/4 (December 2024).
Interview with the winners of the third prize
Firstly, we will provide some information on the winners of the third spot:
Simon Rommelspacher has been with the Federal Statistical Office of Germany since 2016. He worked seven years in the Business Register department on profiling of enterprises and enterprise groups. He then became the Head of the Structure of Trade and Services and Business Statistics section in March 2024. Simon holds a Master’s degree in Business Ad-ministration from the University of Marburg. He also studied computer science at the Uni-versity of Marburg.
Adrian Urban has been a Research Assistant in the Statistical Business Register section of the Federal Statistical Office of Germany since 2022 and a Statistician since May 2024, working on enterprise groups in both roles. He completed both his M.Sc. in International Economics and Public Policy and his B.Sc. in Economics at the University of Mainz.
SJIAOS: As a starter for this interview, can you both tell us why you chose to join the Federal Statistical Office of Germany (Destatis)?
Simon: Statistics was already my main focus during my Master's degree and I also wrote my Master's thesis on bootstrapping time series data at the Statistics Department of the University of Marburg. My statistics professor, Karl-Heinz Fleischer, inspired and encouraged my enthusiasm for statistics. I then applied for a job at the Federal Statistical Office in the business register and was able to contribute to the profiling of enterprises and the maintenance of enterprise group data. For me, official statistics are essential for a modern democratic society and I am very proud to be part of it and to contribute to the high quality of official statistics.
Adrian: During my master studies in International Economics and Public Policy at the university of Mainz, I realized that I was particularly interested in topics related to statistics. I especially enjoyed working with data in statistical software. Given that the Federal Statistical Office of Germany has access to large data sources and offers opportunities to work extensively with data and contribute to providing data relevant for policy decision-making, it quickly became clear to me that a position at Destatis would be a perfect fit. Therefore, I pursued a career at Destatis immediately after completing my studies. Now, having been with Destatis for over two years, I am very glad to have taken this path.
Dear Simon and Adrian, please accept my warmest congratulations on this prize.
SJIAOS: Your prize-winning manuscript is on RUMS – how to compare structures of enterprise groups? Can you tell us a bit more about the content and the motivation for this specific research?
Simon and Adrian: The idea for our similarity measure RUMS emerged in early 2023 when we were discussing the continuity of enterprise groups within our team. This discussion was triggered by changes we made to the way enterprise groups are maintained in the German Statistical Business Register.
In this context, we needed to determine how to ensure the continuity of enterprise groups in the German Business Register moving forward. It was crucial to ensure that each enterprise group is updated with the correct information for the new reference year, thus maintaining continuity for as many groups as possible.
We recognized the need for a method to decide how enterprise groups should be updated for the new reference year. This led to the development of RUMS, which originally aimed to make enterprise groups within the German Register comparable between different reference years and to aid in the decision-making process for updating them.
Later, we realized that RUMS could also be used to measure the similarity of enterprise groups in the German Business Register and in the EuroGroups Register (EGR). This broader application added further motivation to our research, as it could provide valuable insights for both national and international statistical practices.
SJIAOS: What were the main challenges you experienced in doing this research?
Simon and Adrian: One of the main challenges we faced during this research was validating that equal weighting provides the optimal weights for the various parameters in our RUMS. To address this, we conducted extensive simulation studies and applied a geometric procedure to analyse the effects of different weightings, which required significant computational resources and time. For the methodological and mathematical development of these ideas, we are very grateful to Martin Beck, with whom we were able to discuss new ideas and their implications.
Another major challenge was making sure our statistical model was both accurate and useful in practice. Developing the RUMS involved complex modelling and constant adjustments to get reliable results. We had to ensure our methods were not only correct in theory but also practical and easy to use.
Finally, time constraints were also a significant challenge. It took a considerable amount of time to develop a final, satisfactory RUMS formula and to apply it to our data.
We were able to overcome these challenges because we had excellent support from our team and our superiors. We would like to take this opportunity to thank Roland Sturm in particular. Through continuous technical discussions and critical reviews, we were able to work with a high level of motivation and develop new ideas for the RUMS.
SJIAOS: And how were you informed about the YSP prize and what finally stimulated you to write the paper?
We heard about the YSP through an article on our intranet. Since we already presented the idea and a first version of the RUMS in other meetings with a statistical context, the possibility to further develop the RUMS and to have the chance to receive an evaluation from the IAOS award committee motivated us to write the paper. Additionally, we were highly encouraged by our team colleagues and our supervisor to take this opportunity, which gave us even more motivation.
SJIAOS: Could you describe what profiling of enterprises in enterprise groups for business statistics is about, and how is it put in practice?
Simon and Adrian: Profiling of Enterprises is a method to analyse the legal, operational and accounting structure of an enterprise group on a national and a global level in order to delineate the statistical enterprises within the group. The enterprises delineated in this way are the units of presentation for the structural business statistics.
A good quality of data in the business register on the structure of all enterprise groups is needed to carry out profiling (manually or automatically). RUMS helps to achieve a good quality of this data.
SJIAOS: Has your methodology been implemented yet by Destatis or is it going to be in a near future?
Adrian: Yes, the RUMS methodology has been successfully implemented by Destatis. It is used right now for updating the enterprise group data to the next reference year (2023). Additionally, RUMS is used for comparing the enterprise group data of our national business register to the EuroGroups Register (EGR). Here, we use it to manage the manual treatment of the most important groups.
SJIAOS: Why is it so important to have enterprise group data in the business registers, how are they used in national official statistics?
Simon and Adrian: Business registers containing enterprise group data are important for creating an accurate and detailed picture of economic activities and structures. Enterprise groups are becoming increasingly important in the global economy and their structures are becoming more and more complex. We consider it very important to reflect this reality in our data and statistics and to make them available to society, research and politics.
SJIAOS: How do you see the main challenges of building Business Registers in the European context/How to you collaborate with Eurostat or with other National statistical offices?
Simon: In the context of enterprise groups, Germany and the other National statistical offices of the EU and EFTA countries collaborate with Eurostat by sending data on multinational enterprise groups annually. Eurostat then combines and consolidates all these data to build the EuroGroups Register. Since this is a very complex procedure, a considerable amount of time is needed for the consolidation.
One main challenge in this context is the alignment between the data on multinational enterprise groups in the EGR and in the National Statistical Business Registers. Ideally, both registers should have the same numbers of multinational enterprise groups with the same group structures, especially with regard to the national parts. However, due to the complexity of the data consolidation and the fact that the data come from different business registers of various countries, the numbers of groups and the structures deviate between the registers in multiple occasions.
SJIAOS: What is the impact of European regulations (esp. European Business Statistics/EBS) on the work on enterprise groups and how do you see the importance of this subject in the future?
Simon and Adrian: European regulations with regard to European Business Statistics aim to ensure that business statistics are accurate, reliable, and comparable across member states. This accuracy and comparability are very important for a good knowledge base, which official statistics should create for society and research, and we still have a lot to do to achieve this.
Thank you for this interview and best wishes for a successful career.
Interview conducted by Jean-Pierre Cling in July 2024
The winning manuscript will be published in SJIAOS Vol 40/4 (December 2024).
Interview with the winners of the special commendation for a paper from a developing nation
Dear Carmelita, Chelsea and Gabriel, please accept my warmest congratulations on this prize.
SJIAOS: As a starter for this interview, can you both tell us why you chose to join the Central Bank of the Philippines (BSP)?
Carmelita: As a data scientist and seasoned civil servant from the National Economic Development Authority (i.e., Ministry of Economics in the Philippines), I chose to join the Bangko Sentral ng Pilipinas (BSP) (i.e., Central Bank) to harness my analytical skills and policy expertise to help advance data-driven decision-making, shape monetary policies, and drive innovative strategies in promoting financial stability.
Chelsea: I chose to join the BSP because I have always wanted to contribute to the greater good while having opportunities for professional growth. As a data scientist, I tackle problems from an analytical standpoint which gives another dimension to the traditional approach the seasoned central bankers offer.
Gabriel: I have always wanted to become a civil servant ever since considering a career path. The opportunity to work in an organization where I can make a meaningful impact through public service inspired me to pursue a career at the BSP, which is dedicated to improving the lives of Filipinos.
SJIAOS: Your Special Commendation Award-winning manuscript is on E-Commerce Price Index Prediction with Time Series Mining and Automated Machine Learning. Can you tell us a bit more about the content and the motivation for this specific research?
Carmelita, Chelsea and Gabriel: The research aims to overcome the limitations of traditional price index methods by utilizing near real-time data from e-commerce platforms, which offer timely insights into price fluctuations compared to survey-based approaches. The study extracts significant trends from e-commerce data by employing a pipeline of Time Series Mining and Automated Machine Learning to generate an alternative price index. As an initial step, we developed an alternative e-commerce index focused on food commodities to pioneer the use of e-commerce data and advanced analytics to modernize how we understand the current price trends.
SJIAOS: What were the main challenges you experienced in doing this research?
Carmelita, Chelsea and Gabriel: The three main challenges that we encountered were the following :
- Data Quality and Variability: E-commerce data can be vast and heterogeneous, posing challenges regarding data quality, completeness, and consistency. Ensuring that the data used for analysis is accurate and representative was a significant hurdle.
- Complexity of Price Dynamics: Prices in e-commerce platforms can exhibit complex and volatile patterns influenced by factors such as promotions, seasonality, and market dynamics. Capturing and modeling these intricate price behaviors was challenging.
- Model Selection and Optimization: Implementing Time Series Mining and Automated Machine Learning techniques required careful selection and tuning of models to achieve robust and reliable predictions. Balancing model complexity with interpretability was another consideration.
SJIAOS: And how were you informed about the YSP prize and what finally stimulated you to write the paper?
Carmelita, Chelsea and Gabriel: Upon receiving the email about the YSP prize, Mr. Rossvern Reyes, our former supervisor, encouraged us to submit a paper. Since the formulation of the BSP Big Data Roadmap in 2019, the development of a higher frequency CPI has been in the pipeline because the BSP recognized that using big data will aid in monitoring volatile price changes. Also, as with any roadmap, it serves as a guide in operationalizing the use of big data as well as its related activities, e.g., information technology (IT) infrastructure building, in the BSP. In 2023, we consulted the Philippine Statistics Authority (i.e., the Statistics Agency) on CPI generation, collaborated with the Department of Trade and Industry for the data generated in their electronic platform, and gathered enough datasets from private companies with e-commerce data for a pilot run. It is also timely that we learned about the YSP prize since submitting it to the IAOS could help us further review and improve our models.
SJIAOS: Did you experience good coaching and support from your team and management?
Carmelita, Chelsea and Gabriel: Definitely! Our team works collaboratively, where we draw on each other’s strengths. Meanwhile, our Senior Director, Mr. Redentor Paolo Alegre Jr., always ensures that our outputs are of high quality and value and his insights helped us to draft our paper better.
SJIAOS: Can you give us more details on the predictive part of your work? More specifically, predicting the one-month ahead CPI
Carmelita, Chelsea and Gabriel: The main objective of the research is to generate a composite e-commerce price index (CEPI) using e-commerce data and advanced predictive modeling techniques. Further, the CEPI is a composite index derived from e-commerce price data, reflecting the overall price levels of goods sold online.
This analysis helps understand which products or categories within e-commerce data contribute most significantly to predicting changes in the food CEPI, that mostly coincide with the changes in the traditional food CPI. We underline the importance of specific commodities, such as coffee products, in forecasting the food CEPI.
SJIAOS: Some other initiatives have been launched internationally, using data mining to produce a CPI, such as the Billion Price Index conducted by the MIT and Harvard University. Are you aware of these other projects, and how would you compare your work with theirs, in terms of methodology and predictive results?
Carmelita, Chelsea and Gabriel: There are some similarities in our paper with the Billion Price Project (BPP) in that we are both trying to construct an alternative CPI using online data. However, the BPP utilizes standard CPI methodologies and official category weights while our study leverages various machine learning algorithms in deriving the CEPI. In terms of correlation with the official CPI, our models are at par with the models in the BPP. Our alternative CPI has a positive correlation of 90.75% with the National CPI.
SJIAOS: How do you think your research might be used by the Central Bank in the near future or medium term?
Carmelita, Chelsea and Gabriel: In the medium term, the generated pipeline introduced in the study could also serve as a building block in the development of a higher frequency CPI that could help the BSP in monitoring changes in prices in near real-time.
SJIAOS: Most of the competitors in the YSP work in National statistical offices, whereas you are employed by the Department of Economics and Statistics of the Central Bank. Is your department in charge of producing and releasing official statistics?
Carmelita, Chelsea and Gabriel: Yes. The Department of Economic Statistics (DES) is the major data producer of the BSP. The DES-produced statistics can be divided into three categories:
- External Sector Statistics. This includes the Balance of Payments and International Investment Position statistics, Gross International Reserves, Overseas Filipino remittances, and Foreign Direct Investment.
- Monetary and Financial Statistics. This includes reports on the financial linkages and interdependencies of the domestic economic sectors with the rest of the world, such as the Balance Sheet Approach and the Flow of Funds .
- Expectations Surveys and Leading Indicators. These include reports and surveys focusing on forward-looking statistics or leading indicators of economic activities in the country, such as the Business and Consumer Expectations Survey, Consumer Finance Survey, and Residential Real Estate Price Index.
SJIAOS: How do you see the future of big data in the work of the Department of Economics and Statistics of the Central Bank?
Carmelita: The future of big data in the DES of the BSP holds tremendous potential for transforming economic analysis and policy-making processes. Building upon the BSP Big Data Project launched in October 2019, which leverages high-frequency data sources and cloud-based big data and machine learning initiatives, the Department is poised to enhance its capabilities significantly. These advancements will enable more granular and real-time insights into economic trends, improving the accuracy and timeliness of economic indicators used for policy formulation. Furthermore, the formulation of robust big data governance policies ensures that data privacy, security, and ethical considerations are rigorously maintained, fostering trust and compliance in data-driven decision-making. Looking ahead, continued innovation in data analytics within the Department will likely lead to more adaptive and responsive monetary policies, better risk assessment frameworks, and a deeper understanding of economic dynamics in an increasingly interconnected and digital world. As the scope and volume of data continue to expand, the DES is well-positioned to harness these capabilities to maintain its leadership in shaping effective economic policies and strategies.
SJIAOS: Can I ask you the same question about the future of Machine Learning in the work of the Department of Economics and Statistics of the Central Bank?
Chelsea: The future of Machine Learning in the DES of the BSP is indeed promising, specifically in generating alternative statistics to support and complement traditional economic indicators. The Department has several initiatives involving machine learning, which include constructing the News Sentiment Index and identifying key predictors of Real Estate Prices. These innovations underscore the Department’s commitment to leveraging advanced analytics to deepen economic insights and improve forecasting accuracy in an increasingly data-driven landscape. In the coming years, we expect to see more innovations leveraging ML that are geared toward delivering our mandate.
Thank you for this interview and best wishes for a successful career.
Interview conducted by Jean-Pierre Cling in July 2024
The winning manuscript will be published in SJIAOS Vol 40/4 (December 2024).