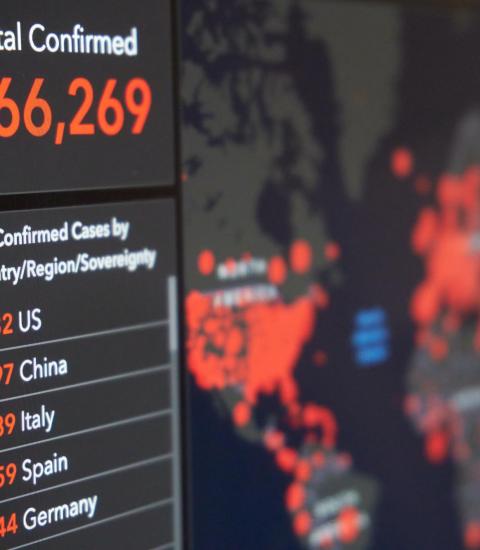
The first set of statements in the special discussion on the SJIAOS discussion platform focused on the roles of Official Statistics in the context of the COVID-19 crisis. It stated the important role that official statistics will have to play in the phase of world-wide recovery from the pandemic and the rapid investments and actions that are needed to fulfill properly this role. With this second set of statements we want the discussion to focus now on the impact of the pandemic on statistical methodology and especially the effect the of the strong economic downturn on techniques and time series data used for estimations in now- and forecasting.
Second set of statements: Official Statistics in the context of the COVID-19 crisis; Official statistics methods need investments to be robust enough to maintain sufficient product quality in times of economic downturns.
This discussion on the SJIAOS discussion platform is based statements extracted from the article ‘Robust official business statistics methodology during COVID-19-related and other economic downturns’ by Paul A. Smith and Boris Lorenc (respectively. University of Southampton; Bright Lynx Research; European Network for Better Establishment Statistics). This article is available as blog on https://officialstatistics.com/news-blog/robust-official-business-statistics-methodology-during-covid-19-related-and-other.
Main statement for discussion:
Official statistics methods need investments to be robust enough to maintain sufficient product quality in times of economic downturns.
Official statistics has not properly researched and understood how its methods and models behave at times of downturns (and potentially in the corresponding situation of similarly paced (unpredictable and fast) growths – though these seem to be infrequent compared to downturns). There is generally a wish to make methods robust to unusual changes, but these are often tackled situation by situation. Production of official statistics during COVID-19 has necessitated some radical changes in both data collection and statistical methods; these have been introduced with admirable speed and dedication, but this process would have been made easier with a body of research already in place to draw from.
Other subsidiary statements for discussion:
Models and assumptions are tuned and trained according to ‘average behaviour’, and under-represent periods of downturn.
Methodological investigations often use recent periods of data in testing. These may not include a period of downturn at all. If they do, such periods are a minority of observations, so they have a small impact on decisions. Strategies are needed to ensure that decisions are effective and methods are robust to many situations.
Many data science approaches are predicated on finding relationships among variables and using them as the basis for statistical outputs, and these are clearly susceptible to changes in those relationships. These procedures also need robustness to be effective when situations are unusual.
At first sight it may seem that different methods or models should be used in downturns to cope with the changes in relationships. However, this would require a crystal ball to know when, for example, a downturn was happening (or about to happen), to know when to change methods. Even determining which are the right periods to upweight in a training data set is not obvious…what is the reach of a downturn?
The current COVID-19 related downturn is an opportunity to gather data which can form the basis of further research into robustness of different methods and stability of relationships between different variables.
It would be helpful to distinguish between intrinsic (‘causal’) and statistical relations, as the former should be more robust to changes. Now there is an opportunity - not existing in 'normal' times - to gather empirical evidence and learn. To further their knowledge of business operations, survey methodologists are likely to benefit by collaborating with business economists and experts in business functions.
Research on the way methods perform in measuring downturns is not sufficiently prioritised
Interest in measuring downturns is strongest when we are in one, as then official statistics outputs are of particular and wide interest. But efforts during a downturn are focused on outputs, not methodological investigation. Once a downturn has ended, the priority is reduced and investigations are not followed through, so research on the performance of methods during downturns is sparse.
AvanDelden
Thanks for initiating this useful discussion with respect to the effects of COVID-19 on the methodology producing official statistics. At Statistics Netherlands, we tried to anticipate which effects an economic downturn due to COVID-19 might have for the production of different business statistics. Such an analysis was not available yet at our office. I was involved in a group looking into effects on the short-term business statistics (STS). We looked into different processes of making the STS, from input (the response fractions), throughput (editing, imputation, analysis) to output (e.g. making timelier output). We worked on various adaptations, for instance on the editing an imputation process. So far, we were using a score function for selective editing where units with a large or small growth factors (period-to-period, or year-on-year) lead to extreme values of the score function; those units are passed on to manual editing. However, in COVID-19 many businesses had a an extreme growth rate and therefore this score function was not so informative anymore. We therefore developed a new, alternative score function. We also made adaptations to other processes. For instance we computed alternative imputation values, making use of a newly available data source. Furthermore, we intensified our analysis stage which is used to validate the results: we added a large number of checks on the outcomes. From 14-17 September 2021 there was an European Establishment Statistics Workshop (EESW21) where this work was presented. The programme and the abstracts can be found at https://statswiki.unece.org/display/ENBES/EESW21+Programme, the accompanying papers will be available by end of October.
I believe that it would have been very useful when experiences from different countries / NSIs were available on the robustness of methodological steps to economic downturns and on how to improve this robustness. That could assisted NSIs to make their processes more robust. To give an example of this usefulness, at the same EESW21 workshop there was a session on the effect of COVID-19 on seasonal adjustment. Different NSIs were struggling with similar issues and the exchange of ideas of how to make use of certain types outliers to improve the seasonal adjustment was very useful.
The COVID-19 crisis was not the first economic downturn and it will very likely not be the last one. It would therefore be very useful when more research is being done in how various methodological steps can be made more robust for the more extreme situations.