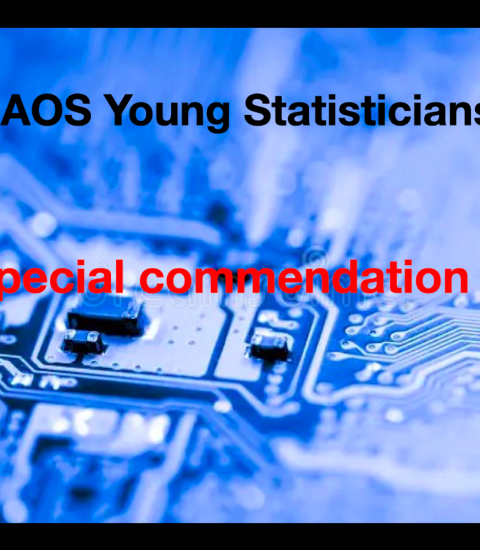
Dear Natalie, Benjamin and Ian, please accept my warmest congratulations on this prize.
SJIAOS: As a starter for this interview, can each of you tell us why did you choose to join the Hong Kong Census and Statistics Department?
Natalie: I obtained B.Sc. and M.Phil. in Statistics from The Chinese University of Hong Kong and discovered my passion for data analytics and statistical programming. During my undergraduate study, I had an opportunity to work as a summer intern in the Census and Statistics Department. This internship experience deepened my understanding and interest in official statistics, and led me to choose to join the Census and Statistics Department after graduation.
Ian: Both my Bachelor's degree and M.Phil. degree are in Statistics. After graduation, I have always been hoping to be a civil servant as I enjoy serving people with my expertise. Moreover, my work offers me a lot of opportunities to do research and attend different courses to enrich my knowledge. The enjoyable working environment made me choose my future career path in the Census and Statistics Department.
Benjamin: I received B.Sc. and M.Phil. in Statistics from The Chinese University of Hong Kong. After graduation, I worked as a data science practitioner in the private sector. In February 2022, I joined the Census and Statistics Department as a Research Manager. With my background in statistics and data science, I aspire to drive data analytics in official statistics. I am fortunate to work on this big data project related to merchandise trade.
SJIAOS: Your prize-winning manuscript is on a risk assessment approach for misclassifications using deep-learning techniques. Can you tell us a bit more about the content and the motivation for this very specific research?
Authors: Over the years we have been exploring the application of deep learning techniques to process trade declarations, such as performing text analytics on free-text commodity descriptions and building deep learning models based on a host of data fields for more comprehensive quality assurance. With the recent advancements in text analytics methodology and computing power (e.g. use of GPUs), the 20 million trade declarations received each year provide a plentiful database for us to mine useful patterns and draw insights through the application of deep learning techniques. Through the models being developed, we can model the information reported on a trade declaration (such as commodity code, quantity and total value of goods) based on the free-text commodity descriptions and perform quality checking on the trade declarations.
SJIAOS: What were the main challenges you experienced in doing this specific research?
Authors: The challenges in doing our research came from the heavy demand of domain knowledge, technical skills and computing resources.
First, there were data quality issues, especially related to the free-text commodity descriptions. We had to tailor-make text preprocessing steps and adopt suitable models based on our domain knowledge.
Second, deep learning is a rapidly evolving field with new techniques emerging all the time. We had to keep abreast of the latest developments and train statistical staff continuously so as to progress with the times.
Lastly, our model training requires powerful computing resources, especially graphics processing units (GPUs). We had to upgrade the hardware and software from time to time and ensure the efficient utilization of computing resources.
SJIAOS: And how were you informed about the YSP prize and what finally stimulated you to write the paper?
Authors: We were informed about the YSP prize by our internal communication. The encouragement from our supervisor and the directorate of the Census and Statistics Department stimulated us to write the paper.
SJIAOS: What is the role of your branch (Trade Statistics Branch (1)) in the Census and Statistics Department?
Authors: Trade Statistics Branch (1) is mainly responsible for verifying the information reported on trade declarations and compiling and disseminating the monthly external merchandise trade statistics and trade index numbers of Hong Kong. Also, our branch will conduct research on the application of big data analytics on trade data, and perform enhancement and maintenance on the big data analytics models.
SJIAOS: Natalie:. You are a Statistician at the Trade Analysis Section. Can you explain in some detail what your function in the section is and what your specific role in the prize-winning paper research has been?
Natalie: As the head of the Trade Analysis Section, my primary role is to oversee the compilation and dissemination work of the monthly external merchandise trade statistics and trade index numbers of Hong Kong and to ensure a timely and accurate provision of trade statistics. I also monitor trade statistics at a macro level and provide statistical support to external parties to facilitate policy formulation and economic analyses. In this research paper, my role is to apply the text analytics model to the existing quality assurance mechanism for trade declarations to facilitate the compilation and enhance the quality of trade statistics.
SJIAOS: Ian for you the same question as for Natalie, but I understand your role in the research to be specifically directed to the Big data part?
Ian: Yes, Trade Research and Analytics Section (1) is established in April 2023. It is mainly responsible for identifying and investigating anomalies on the data in trade declarations and developing big data analytics models to verify the accuracy of information reported in them. Moreover, I am responsible for conducting quality assurance of aggregate trade statistics before it is published.
SJIAOS: Benjamin: your job is Research Manager at Trade Statistics Branch (1). I understand that you also were responsible for the invention of the unit value model and the total value model of the project. Could you explain a bit in more detail what the fundamental role of the two models in your research is?
Benjamin: The unit value model and the total value model constitute integral parts of our data quality assurance mechanism. They are used to verify the declared quantity and total value of goods reported on a trade declaration, which is then essential to the accuracy of merchandise trade statistics. Currently, these two data fields are verified by a traditional rule-based model and suspicious cases are filtered for further manual checking. These two models, which are still under development and testing, are constructed by adopting probabilistic deep learning, which is a modern idea related to the prediction of a distribution by deep neural networks.
SJIAOS: Machine learning and deep learning are with the development of AI in our day-to-day life important features of modern applied statistics. How do you personally and in general how in Statistics Hong Kong is AI already a regular part of the statistical program?
Authors: We think AI is already a regular part of our work. Our duties include utilising the data to find patterns, automating the working procedures and building a programming-based architecture for our daily routine. In general, AI has become more popular in Hong Kong. We can see chatbots in different websites and apps, and the trend of mentioning AI in various industries is certainly upward. It is important to note that integrating AI into the statistical program is still an ongoing process in Hong Kong. As AI technologies continue to advance, we, as statisticians in Hong Kong, are responsible for incorporating them further into our daily workflows.
SJIAOS: What do you consider the most challenging features of deep-learning methodology, in statistics in general and especially in the domain of trade statistics?
Authors: In general, the training of a deep learning model requires large amounts of labeled data. Otherwise, it is prone to overfitting. Acquiring and preparing such data can be costly and time-consuming.
In Hong Kong, traders are required to lodge declarations to the Hong Kong Government within 14 days after the importation or exportation of an article, except those which are exempted to do so. The availability of 20 million declarations received annually makes the deep learning approach feasible. A challenge is that we need to ensure this approach is sustainable and robust to changes in the data. Note that the Harmonized System (HS) is a commodity classification system regularly updated by the World Customs Organization (WCO). Since the HS and the unit values of commodities change with time, we have to constantly update the models to ensure they are valid for data quality assurance.
SJIAOS:… and what are your expectations for the use of the deep-learning methodology in the next few years? What kind of challenges, risks and opportunities do you see in this domain?
Authors: The use of deep-learning methodology will continue to grow rapidly in the next few years, driven by the increased availability of computing resources and enhanced technology. Also, models with enhanced interpretability will be seen in the future.
One of the challenges is the need for more training data. As we all know, an excellent deep-learning model usually requires a large amount of cleaned (and even labeled) data. We need to collect and process these data, which may be time-consuming and expensive.
There are concerns about bias and ethical issues in deep learning models. Bias in training data can lead to biased predictions and unjust conclusions. We should address these problems and make our best effort to ensure the deep learning models are transparent and unbiased in the future.
Regarding opportunities, deep learning models can automate complex tasks that previously required heavy manual work, just like how our deep learning models do. It can increase efficiency, reduce costs, and improve productivity in various sectors.
SJIAOS: With all the recent new developments in deep-learning, ML, AI, and the ecosystem of (also small area) data, how do you see the production and dissemination work of the national statistical office being organized in 10-15 years?
Authors: In the coming 10-15 years, we believe that the new developments in deep-learning, ML, AI can facilitate the automation of data collection, processing, and analysis. National statistical offices may incorporate diverse data sources, such as social media and satellite imagery, alongside traditional survey data to enhance the coverage, timeliness and accuracy of statistical outputs. The dissemination of official statistics are also likely to evolve, with interactive visualizations and user-friendly interfaces facilitating better data understanding.
The winning manuscript will be published in SJIAOS Vol 39/4 (December 2023).
It was a pleasure to have this interview with you and I wish you success in your further career.